
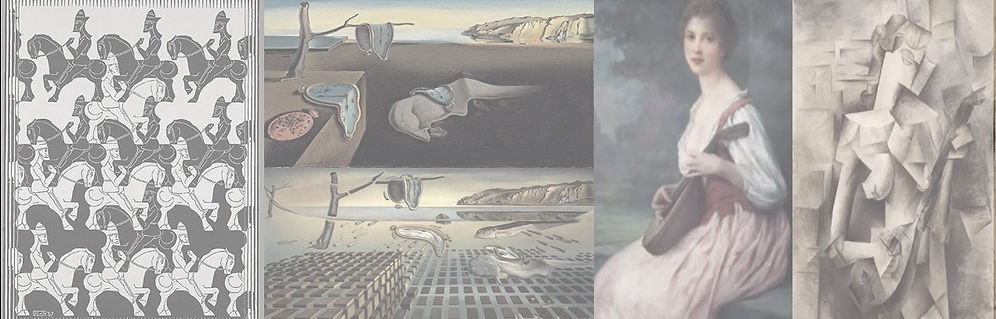
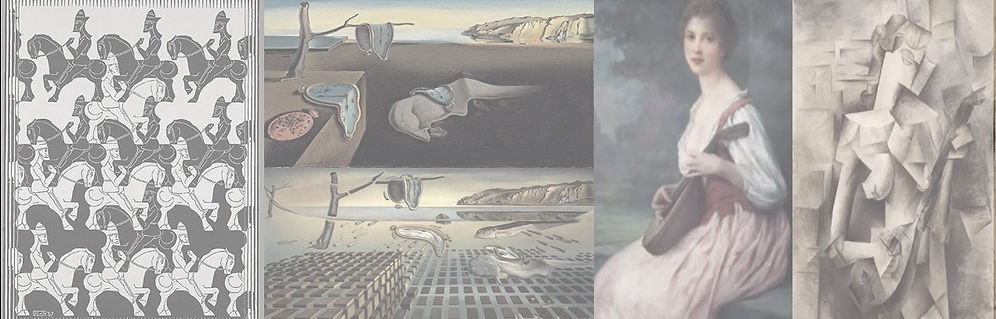
All my life through, new insights on nature made me rejoice like a child!
Marie Curie
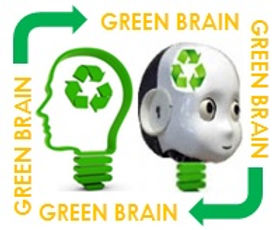
Intelligent recycling of existing functions to support newer (more complex) functions while building complex systems (software, chips etc.) often leads to viable designs that are space, connectivity and energy efficient.
But is “Brainlike Intelligence” itself an outcome of efficient recycling in the first place?
GREENBRAIN is a new direction I am presently developing , that both connects and takes forward my work in the field of action, skill, affordances, memory and social cognition. In short, GREENBRAIN is a comprehensive investigation of the principle of “Recycling” both as a fundamental necessity in any complex viable design (in terms of conserving space, time, energy) and a fundamental recurring principle across domains (perception-action-memory-
reasoning-social intelligence) in any system (Natural/Artificial) capable of producing cognition. Such an investigation is the need of the time given both a) emerging trends from neurosciences, connectomics indicating cross domain functional recycling at sensory, motor and cognitive levels in the brain [1-9] and b) The still inherent difficulties while building cognitive robots, assistive agents capable of exhibiting brainlike intelligence in the complexity of the environments we inhabit and create [10,11]. Both these domains presently mandate the need to look for novel, unifying principles given that a central challenge for both brain science eengineering today is to “causally” and “computationally” correlate the incredibly complex behaviors of animals to the equally complex activity in their brains, create better cognitive machines, robots that can meaningfully assist us in the environments we inhabit and create, given the increasing complexity of our society and economy.
Further, with several large scale brain initiatives that are generating data at an unprecendented level, the quest to move from data to underleying principles is lagging behind. This is common in the history of science, consider the large amount of star data gathered in astronomy (before that apple fell!) or even the massive data on species (that Darwin looked at to envision the bigger picture). Brain Science today is also at this crucial junture, and one connecting link among data from different domains (Perception-Action-Affordance-Memory-Social cogniton) is the notion of funtional recycling (how different cotrical areas are reused or reactivated in diverse sensorimotor, cognitive and social contexts), quite similar to the component reuse framework of software engineering.
GREENBRAIN in this sense, is a new direction to understand the brain as Recycling Machine: that achieves its diversity of cognitive functions by recycling same regions in a variety of circumstances, putting them together in different patterns of “goal” oriented functional cooperation, to facilitate the survival of a complex body in an unstructured environment.
For the ones, who are interested further,
A 5 year roadmap towards GreenBrain, an initial skeleton of GREENBRAIN research venture subdivided around 21 itnterrlinked tasks, and some recent references that motivate this vision is outlined below. If you find yourself to be a cotraveller in this journey...wish to know more/collaborate/give feed bac do get in touch!!!!!
GreenBrain - Task distribution
GreenBrain roadmap and Task interlinkage (over 60 months)
Top picture shows the mapping of various topics of interest in the GREENBRAIN as organized into 6 potential work packages (covering perception-action, learning, social, architecture, application and managememnt that is not further depicted into subtasks). Bottom panel shows a possible implemnation roadmap over a 5 year period Black, arrows indicate the major interdependencies. In sum this covers also the different areas spannign my work so far and publications and how I intend to take it furhter (in terms of vision, implementation, cognitive architecture software ) and linking it t what we are constantly learning about the brain.
GREENBRAIN References
-
Anderson, M. L. (2010). Neural reuse: A fundamental organizational principle of the brain. (Target article). Behavioral and Brain Sciences, 33( 4), 245– 266.
-
Eichenbaum H, Cohen NJ (2014). Can we reconcile the declarative memory and spatial navigation views on hippocampal function? Neuron. 2014 Aug 20;83(4):764-70.
-
Bressler, S,L., Menon, V. (2010). Large-scale brain networks in cognition: emerging methods and principles. Trends in cognitive sciences Volume 14, Issue 6, June 2010, Pages 277–290
-
Gallese, V., Sinigaglia, C. (2011). What is so special with Embodied Simulation. Trends in Cognitive Sciences. 15(11):512-9.
-
Pickering, M,J., Clark, A. (2014). Getting ahead: forward models and their role in cognitive architecture, Trends in cognitive sciences, 18(9).
-
Allen, T.A. & Fortin, N.J. (2013). The evolution of episodic memory. Proceedings of the National Academy of Sciences USA, 110 (Suppl 2), 10379-10386.
-
T. T. Hills, P. M. Todd, D. Lazer, A. D. Redish, I. D. Couzin, and the Cognitive Search Research Group (2015) “Exploration versus Exploitation in Space, Mind, and Society” Trends in Cognitive Sciences 19(1).
-
Schacter, D.L., Addis, D.R., Hassabis, D., Martin, V.C., Spreng, R.N., Szpunar, K.K. (2012). The Future of Memory: Remembering, Imagining, and the Brain. Neuron, 76, 677–694.
-
Anderson, M L. (2014). After Phrenology: Neural reuse and the interactive brain. The MIT Press.
-
Mohan V, Morasso P (2011) Passive Motion Paradigm: an alternative to Optimal Control. Frontiers in Neurorobotics, Vol. 5, Art. 4, pp. 1-28.
-
Georg Stork., H. (2012). Towards a scientific foundation for engineering Cognitive Systems – A European research agenda, its rationale and perspectives, Biologically inspired cognitive architectures (BICA), Elsevier Science publishers, Vol. 1 (July 2012), pp. 82-91, doi:10.1016/j.bica.2012.04.002.
-
Mohan V, Sandini G, Morasso P. (2014). A neural framework for organization and flexible utilization of episodic memory in "cumulatively" learning baby humanoids, Neural Computation 26(12), 2692-2734, MIT Press.
-
Mohan V, Morasso P, Metta G, Kasderidis S (2011) The distribution of rewards in growing sensory-motor maps. Neurocomputing, 74:3440-55.
-
Hurley, S. (2008). The shared circuits model (SCM): How control, mirroring, and simulation can enable imitation, deliberation, and mindreading. Behavioral and Brain Sciences, 31, 1– 58.
-
Mohan V, Morasso P (2012) How Past Experience, Imitation and Practice Can Be Combined to Swiftly Learn to Use Novel “Tools”: Insights from Skill Learning Experiments with Baby Humanoids. Lecture Notes in Artificial Intelligence, vol. 7375, pp: 1-12.
-
Mohan V, Morasso P, Metta G, Kasderidis S (2011) Actions & Imagined Actions in Cognitive robots. In "Perception-reason-action cycle: Models, algorithms and systems" (Joint Editors: V. Cutsuridis, A. Hussain, JG Taylor), Springer Sries in Cognitive and Neural Systems, vol. 1, Chapter 17, 539-572.
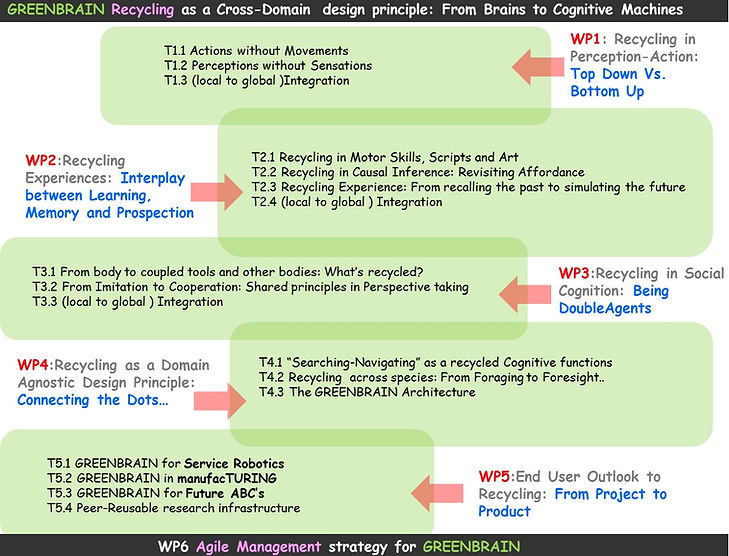
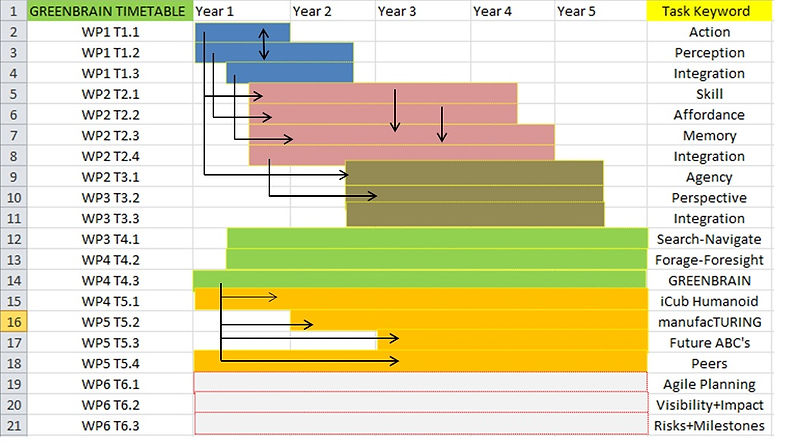